Meet Make Each characteristic Binary: Bing’s sparse neural community for improved search relevance
MEB helps Bing get nearer to look intent by understanding relationships past pure semantics.
Bing has launched “Make Each characteristic Binary” (MEB), a large-scale sparse mannequin that enhances its manufacturing Transformer fashions to enhance search relevance, the corporate announced Wednesday. This new expertise, which is now operating on 100% of Bing searches in all areas and languages, has resulted in an almost 2% improve in clickthrough charge for the highest search outcomes, a discount in handbook question reformulation by greater than 1% and a 1.5% discount of clicks on pagination.
What MEB does. MEB maps single information to options, which helps it obtain a extra nuanced understanding of particular person information. The objective behind MEB appears to be to higher mimic how the human thoughts processes potential solutions.
This stands in distinction to many deep neural community (DNN) language fashions which will overgeneralize when filling within the clean for “______ can fly,” Bing offered for example. Most DNN language fashions may fill the clean with the phrase “birds”.
“MEB avoids this by assigning every reality to a characteristic, so it might assign weights that distinguish between the flexibility to fly in, say, a penguin and a puffin,” Bing mentioned within the announcement, “It will possibly do that for every of the traits that make a chicken—or any entity or object for that matter—singular. As a substitute of claiming ‘birds can fly,’ MEB paired with Transformer fashions can take this to a different degree of classification, saying ‘birds can fly, besides ostriches, penguins, and these different birds.’”
Discerning hidden intent. “When wanting into the highest options discovered by MEB, we discovered it might be taught hidden intents between question and doc,” Bing mentioned.
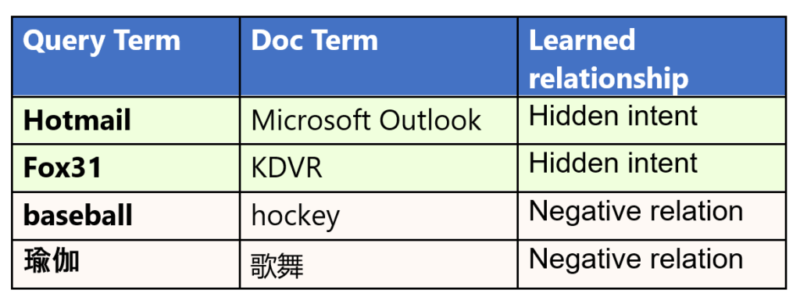
MEB was capable of be taught that “Hotmail” is strongly correlated to “Microsoft Outlook,” though the 2 aren’t shut when it comes to semantic which means. Hotmail was rebranded as Microsoft Outlook and MEB was capable of decide up on this relationship. Equally, it discovered the connection between “Fox31” and “KDVR” (regardless of there being no overt semantic connection between the 2 phrases), the place KDVR is the decision signal of the TV channel that operates below the model Fox31.
MEB may also determine detrimental relationships between phrases, which helps it perceive what customers don’t need to see for a given question. Within the examples Bing offered, customers looking for “baseball” don’t usually click on on pages speaking about “hockey” though the 2 are each in style sports activities, and the identical applies to 瑜伽 (yoga) and paperwork containing 歌舞 (dancing and singing).
Coaching and scale. MEB is skilled on three years of Bing search that comprise greater than 500 billion question/doc pairs. For every search impression, Bing makes use of heuristics to gauge whether or not the person was glad with the end result they clicked on. The “passable” paperwork are labeled as constructive samples and different paperwork in the identical impression are labeled as detrimental samples. Binary options are then extracted from the question textual content, doc URL, title and physique textual content of every question/doc pair and fed right into a sparse neural community mannequin. Bing supplies extra particular particulars on how MEB works in its official announcement.
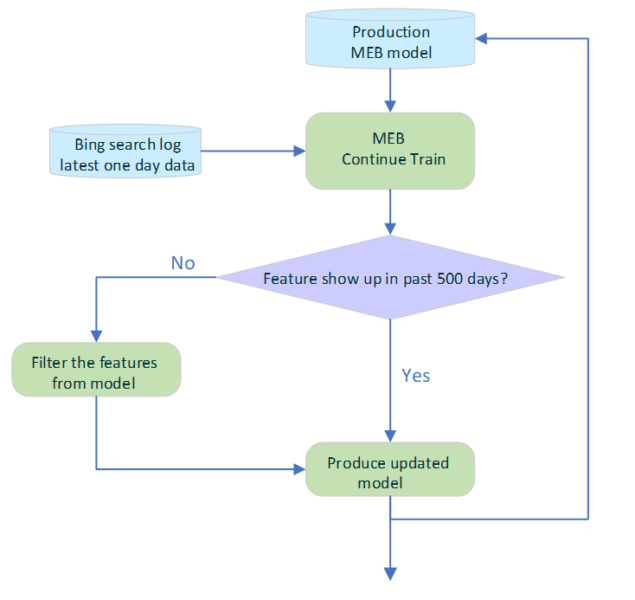
Even after being carried out into Bing, MEB is refreshed every day by repeatedly coaching on the newest every day click on knowledge (as proven above). To assist mitigate the impression of stale options, every characteristic’s timestamps are checked and those that haven’t proven up within the final 500 days are filtered out. The every day deployment of the up to date mannequin can be absolutely automated.
What it means for Bing Search. As talked about above, introducing MEB on prime of Bing’s manufacturing Transformer fashions has resulted in:
- An virtually 2% improve in clickthrough charge on the highest search outcomes (above the fold) with out the necessity to scroll down.
- A discount in handbook question reformulation by greater than 1%.
- A discount of clicks on pagination by over 1.5%.
Why we care. Improved search relevance implies that customers usually tend to discover what they’re in search of quicker, on the primary web page of outcomes, with out the necessity to reformulate their queries. For entrepreneurs, this additionally implies that should you’re on web page 2 of the search outcomes, your content material most likely isn’t related to the search.
MEB’s extra nuanced understanding of content material may additionally assist to drive extra site visitors to manufacturers, companies and publishers, because the search outcomes could also be extra related. And, MEB’s understanding of correlated phrases (e.g., “Hotmail” and “Microsoft Outlook”) and detrimental relationships (e.g., “baseball” and “hockey”) could allow entrepreneurs to spend extra time specializing in what clients are actually looking for as a substitute of fixating on the precise key phrases to rank greater.
For the search trade, this will assist Bing keep its place. Google has already laid out its vision for MUM (though we’re removed from seeing its full potential in motion), and MEB could bolster Bing’s conventional search capabilities, which can assist it proceed to compete in opposition to the trade chief and different serps.